
Computer Vision (CV).
The eyes of innovation powering modern deep tech.
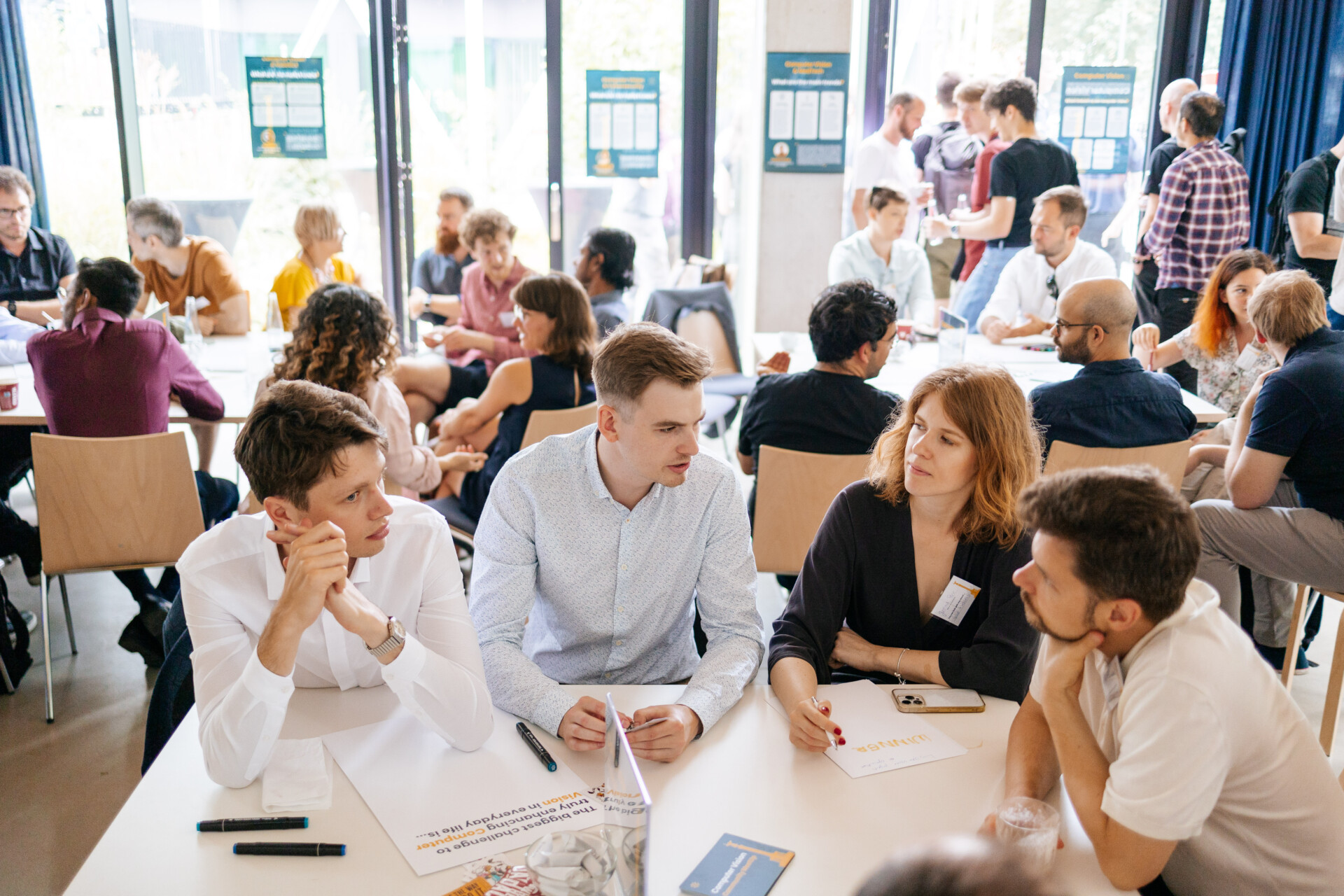
As a leading technology expert, we want to stay updated with the hottest trends. The computer vision field and its progress are among the hottest technology topics. We find it particularly dear to us as we develop groundbreaking solutions in this field. We’ll be happy to assist you with your computer vision-related ideas.
What exactly is computer vision technology?
Simply put, computer vision enables computers and machines to see. However, the reality is far more complex. The method strives to help the devices automatically achieve what the human brain does. It means enabling computers to see and process the images to determine the object’s class and distance and to track them if they’re in motion.
The most common tasks that computer vision wants to achieve include:
Object classification
the ability to put the object in a specific category on sight, just like our brain immediately knows that a dog is an animal.
Object identification
it sounds similar to object classification but goes deeper. It would allow the algorithm to recognise and identify, for example, a specific cat among a larger group of cats.
Object tracking
the ability of a machine to track the object in motion.
Real World Example – Building an in-the-wild gaze estimation solution.
What are the base principles of computer vision work?
Computer vision is a complex technology advancing quickly in recent years. It has also become one of the most important deep tech trends. Computer vision projects involve different adoptions across industries, but how does it work exactly?
Primarily, CV uses pattern recognition techniques to train itself to understand the visual data it receives. The aim is to mimic the human brain and how it processes visual information. Once completed, the object detection process works similarly to human vision. However, it includes the extra finishing step of providing the visual output.
Computer vision algorithms rely heavily on pattern recognition. Initially, the device acts as an eye to receive the input data. After that, the interpreting device (a computer, smartphone or similar) interprets the face recognition data and produces it as an output.
CV devices are trained on large amounts of data to process the images, label the objects present in them and find ways in those objects. The more training data provided, the more accurate the algorithm is and the better its ability to recognise patterns and classify images will be. Ultimately, the goal is for it to identify the object it’s trained in without fail.

What is the future of technology? Let’s learn about Computer Vision!
Applications of Computer Vision
Computer vision in Healthcare
The healthcare industry is one of the first things that comes to mind when discussing image processing. Medical imaging like X-rays, MRI, or CTA scans have been an essential part of computer vision and a game-changer in diagnostics. With the advancements in the field, suggestion systems help determine the correct diagnosis.
Computer vision in Agriculture
Crop and livestock monitoring maximises profits by ensuring uninterrupted growth and safety. Seed selection methods utilise deep neural network models to reject potentially flawed plants. Evaluation of yields using image classification and processing gives automatic insight into the plant’s quality and health.
Computer vision in Robotics and Automotive Industries
The car production industry has been constantly becoming increasingly dependent on cameras mounted in vehicles. Their wide application ranges from parking assistance and pedestrian detection to fully autonomous cars that can detect obstacles with object-tracking features, comply with traffic signs and identify pedestrians.
Key CV trends in Healthcare
Telesurgeries (remote surgeries)
It is expected that advancements in robotic surgery will continue to grow. Some experts predict that robotic surgery will become the standard of care for some procedures. Among other sensors, the system uses advanced computer vision, allowing surgeons to “feel” what they do during the procedure.
Artificial intelligence algorithms fed by specialised cameras will continue integrating into the robotic systems, allowing for more autonomous and precise operations. Virtual and augmented reality advancements will enhance the surgeon’s ability to visualise and manipulate surgical sites. It will also benefit the training before performing actual surgeries.
Understanding of the human body
There is a trend involving the use of deep neural networks to help us understand the functions of the human body. Increasingly sophisticated computer vision methods help to segment blood vessels, identify stroke aetiology, or detect malignant tumours. Complex radiology assistant tools in development are based on advanced computer vision methods.
Health monitoring
By leveraging computer vision techniques, doctors can analyse health and fitness to assist patients in making better medical decisions. In contemporary medicine, doctors use it to gauge blood loss during surgeries, particularly C-sections. It can help take emergency measures more effectively when the quantity of lost blood reaches the last stage. Additionally, this technology can measure the body fat percentage in people using the images captured by regular cameras. The pose analysis algorithm can aid rehabilitation processes and detect postural defects.
![]()
I see the future of computer vision in the medical industry primarily as an opportunity to create systems that assist doctors in their work. In the future, radiologists will not have to annotate medical images manually. A computer vision algorithm will perform the manual work on their behalf, standardising all annotations to a single format. Moreover, it takes 1,000 times less time for an algorithm to describe it manually, so introducing computer visions to hospitals could solve the problem of long waits for radiology results. Reducing this time is an opportunity for patients needing immediate help because, for them, every second less waiting is an increase in the chance of survival.
their work. Once the digital world is entered, the farmlands will become automated, and the whole agricultural production chain will divert to machine supervision.
![]()
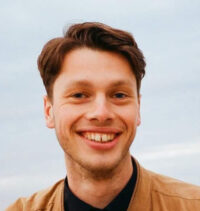
Key CV trends in AgriTech
There are several trends for computer vision projects in the agriculture area.
Monitoring crop and livestock welfare
Installing a fixed camera in an animal stall allows the owner to monitor the livestock remotely. Image processing methods enable automatic anomaly detection like events or mass panic. Computer vision can also aid in ensuring proper animal growth. Instance segmentation and pattern recognition methods allow the automatic tracking of individual animals.
A drone with a camera can help a farmer with monitoring crop growth. Deep learning-based detectors can analyse video data, raise alerts on possible losses, and calculate the waste percentage. Automated monitoring systems can help increase security by automatically detecting intruders or thieves.
Farming assistance
Decision-making systems trained on crop datasets can determine an optimal time window for harvest based on farmland images. A route-planning system with visual aids can aid in managing the fleet, which can save fuel.
Seed and livestock nourishment and yield evaluation
Applying computer vision technology can automatically sort seeds for maximised yield by discarding potentially flawed sprouts. The exact process can be used to select fodder for the livestock and help alleviate health issues caused by spoilt supplies. A computer vision model can also be used to evaluate the uses of deceased animals.
Inspecting harvests can help detect diseases affecting crops and help find a solution to counteract them. Image-supported sorting stands can save manual labour by visually inspecting and automatically separating flawed plants.
![]()
The future of agriculture appears to be strictly connected with the development of computer vision and image processing methods. With the continuous growth of hardware capabilities, miniaturisation and the rise of edge AI, more and more solutions and tools will be within reach of casual farmers. Replacing manual human labour with automated assistance based on computer vision will undoubtedly impact how people in agriculture perceive their work. Once the digital world is entered, the farmlands will become automated, and the whole agricultural production chain will divert to machine supervision.
![]()
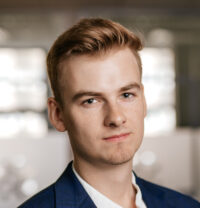
Key CV trends in Robotics
Let’s look at the trends our experts find the most significant and best to carry out a computer vision project.
Development of humanoid robots
Evolving facial recognition and analysis algorithms like emotion recognition, gaze estimation or expression recognition can take human-robot interactions to the next level. With more human-like behaviour, humanoid robots will be able to break the barrier of distrust of machines. CV can give robots reliability, making them an integral part of everyday life.
Mobile robotics and crewless aerial vehicles
For most mobile robots and drones, cameras will replace most currently used sensors. Thanks to computer vision projects, those robots could function with the highest level of autonomy thanks to the intense work of automation experts.
Industrial robotics
Technological processes are another promising field for adopting computer vision projects. Extensive work on simplifying and fine-tuning deep learning models and algorithms can help introduce CV techniques and enable use by industry workers without specialised domain knowledge.
The paradigm of meta-learning can be beneficial in this case, as it tries to mimic the human approach to deep learning model for new tasks by drawing on previous knowledge. Additionally, computer vision algorithms can aid in maintaining the safety of workers, leading to the popularisation of cooperative robots.
![]()
In my opinion, computer vision in robotics will eliminate all other sensors. RGB cameras will be a standalone and sufficient source of information for the systems. Pushing computer vision to the next level is key to obtaining the complete autonomy of mobile robots and UAVs. Increasing the compute power-to-device size ratio will allow for state-of-the-art solutions on the onboard systems. Efforts put into topics like meta-learning will help popularise computer vision in the manufacturing industry even more than they do nowadays.
![]()
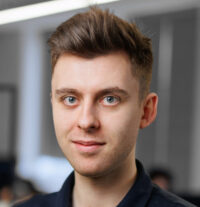
What are the key challenges of computer vision projects?
Our experts name several challenges when it comes to carrying out computer vision projects, and they include:

Running computation-heavy algorithms without the need for a supercomputer

The challenge for autonomous vehicles to identify unusual scenarios like crashed cars, road accidents and other unexpected situations

Object occlusion can pose a challenge to reconstructing a 3D scene e from a series of images. Humans have no problem identifying that two visible parts of a dog standing behind a tree are still the same dog -unfortunately, this is not the case for computer vision algorithms.

Working in dynamic lighting is still a significant challenge for computer vision, making it hard to identify an object in the natural environment correctly.
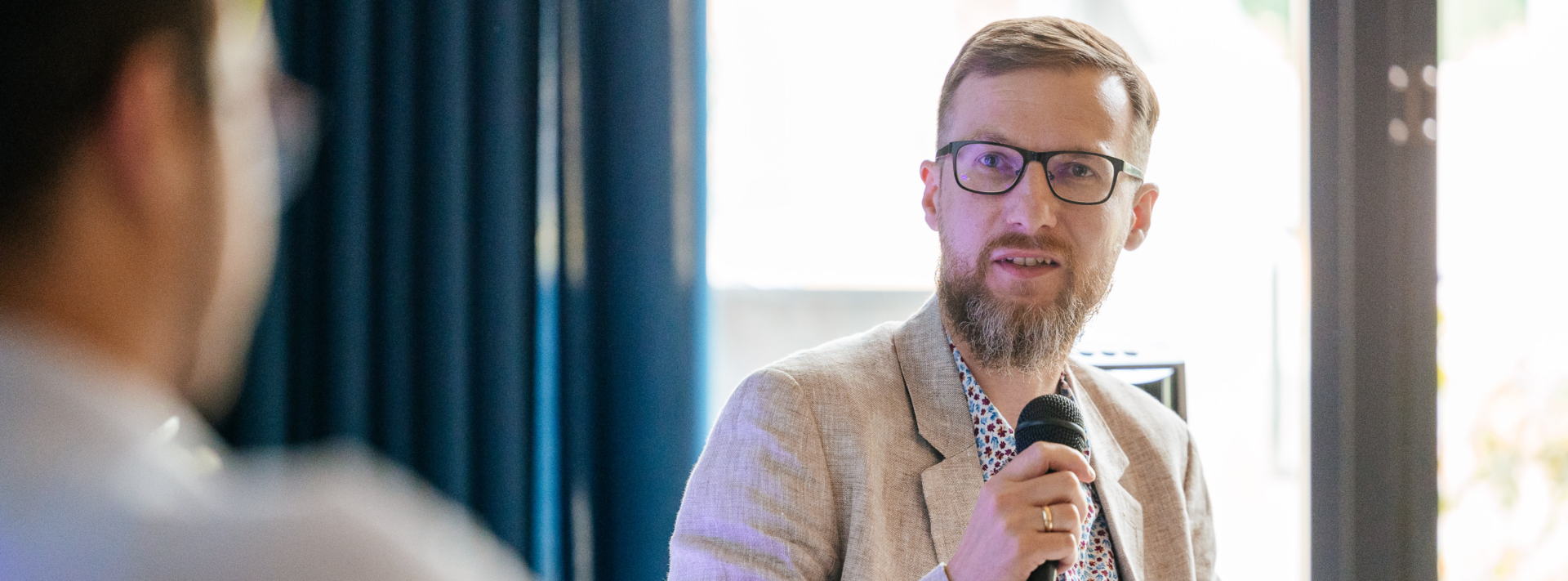
Get to know our computer vision experts:
Marek Tatara, PhD
Head of Science
Assistant Professor at Gdańsk University of Technology, AI/ML Expert at M5 Technology, Member of the Polish Society For Measurement, Automatic Control And Robotics. works on the company’s research agenda and works on the implementation of both EU-funded and commercial R&D projects from the field of Computer Vision, Machine Learning and Embedded Systems.
Jacek Niklewski, PhD
Data Scientist
Involved in projects related to computer vision, sports applications, medical diagnosis, and recommender systems. He graduated from the Computer Science degree in at Gdansk University of Technology. He has a MSc in Investment Management, a PhD in Finance, and a PgCert in Academic Practice in Higher Education at Coventry University.
Karol Duzinkiewicz
Computer Vision Researcher
Seasoned engineer with many years of experience in international tech companies. Currently holds a team leader role in gaze estimation projects developed in the company.
Michał Ostyk
Computer Vision Engineer
A Computer Vision Engineer with experience in agriculture, fast food, sports analytics, and healthcare. He loves researching SOTA and converting it into an MVP in Pytorch. However, he recently delved deeper into MLops.
Jan
Glinko
Glinko
Machine Learning Researcher
Graduated from the Faculty of Electronics, Telecommunications, and Informatics at the Gdansk University of Technology. He is interested in applying synthetic datasets for learning deep neural networks and in learning algorithms to reduce the amount of data required for effective network training.
Michał Affek
Embedded Machine Learning Researcher
Currently enrolled in an industrial PhD programme at the Gdansk University of Technology. His main interests are remote sensing (processing done specifically on satellites), machine learning algorithms for edge devices, and parallel computing.
Cezary
Polak
Polak
Machine Learning Researcher
Graduated from the Faculty of Electronics, Telecommunications, and Informatics at the Gdansk University of Technology. He is interested in using deep learning in biomedical engineering and generating synthetic data such as photos and texts
Artur
Skrzynecki
Skrzynecki
Machine Learning Researcher
Graduated from the Faculty of Electronics, Telecommunications, and Informatics at the Gdansk University of Technology. His areas of interest mainly focus on computer vision tasks, including biomedical data processing and deep neural network training and evaluation. Apart from that, he also enjoys web development topics
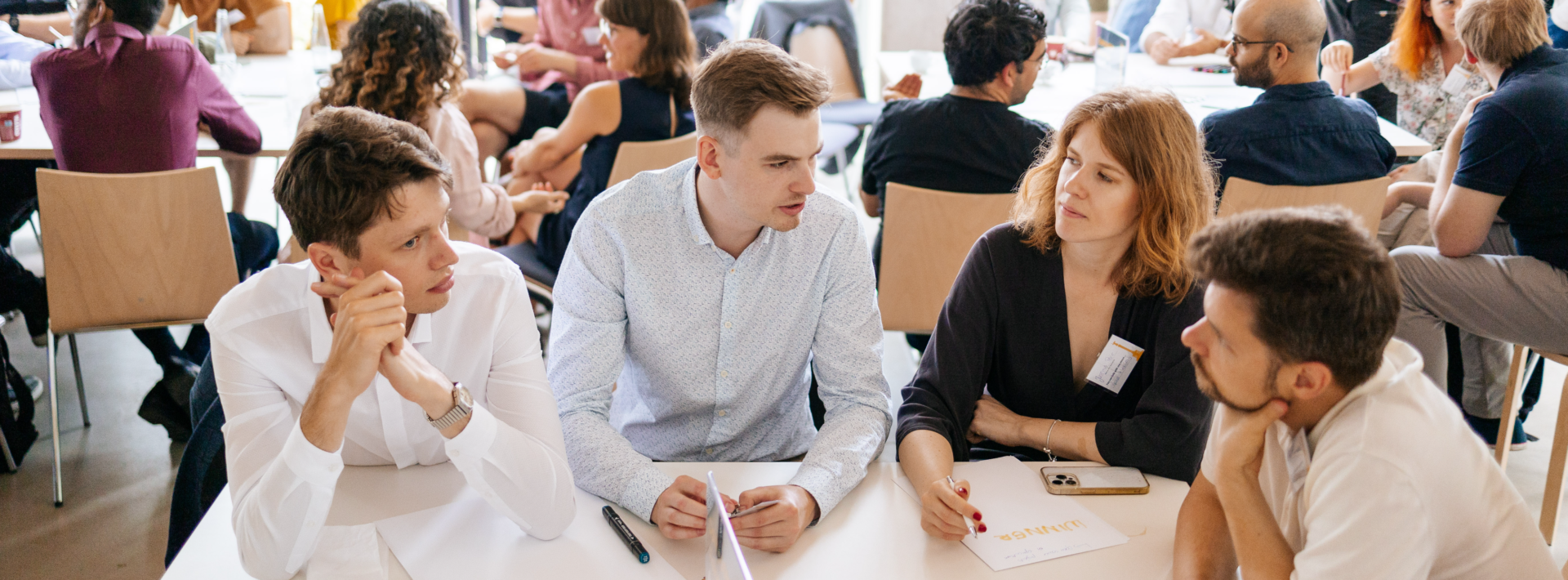
Computer Vision Report
computer vision knowledge in a nutshell – basic information for people who want to get started and gain some insights,
real-life case studies – practical examples of computer vision implementation in various commercial and scientific projects,
trend predictions and future forecasts by experts,
the main challenges computer vision encounters.
Download the report
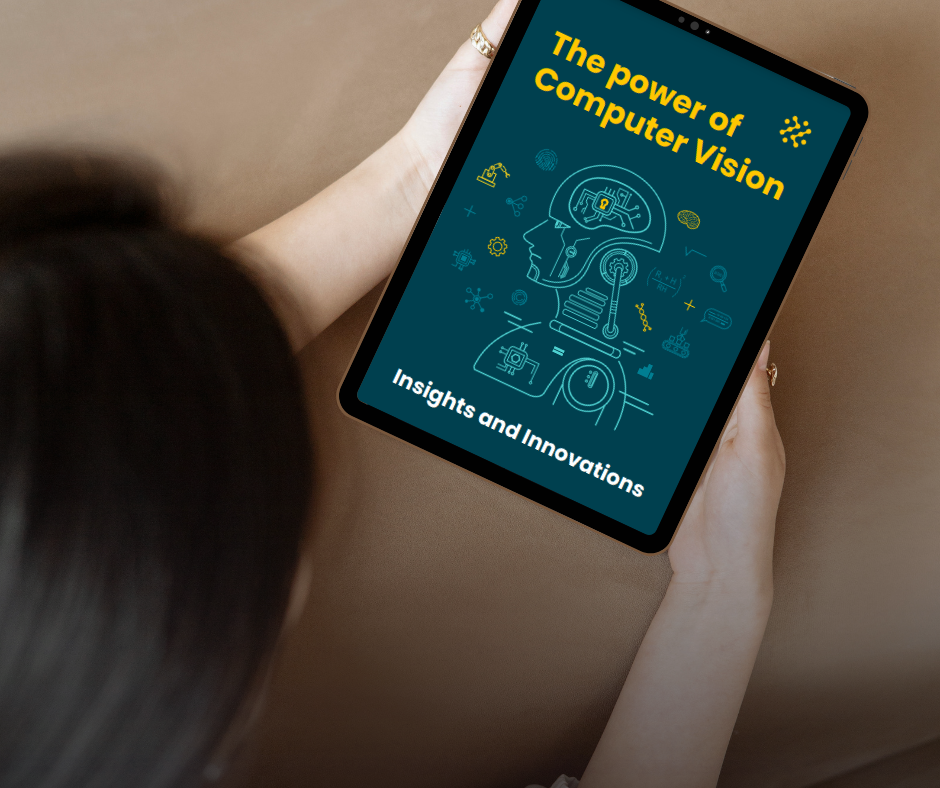
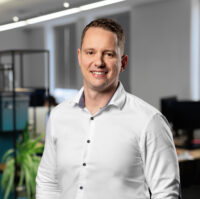
Let’s talk about your project.
Computer Vision and Machine Learning
Naturally, computer vision can only go with machine learning at its very foundation. Algorithms for analysing the input data and their training lie in the very definition of machine learning. It enables the image recognition device to analyse the provided data to increase accuracy.
Therefore, the training data’s amount and quality are crucial.
Interested in Machine Learning?
Check out the topic-related insights


How Can Artificial Intelligence and Computer Vision Enhance the Capabilities of Your Drones?
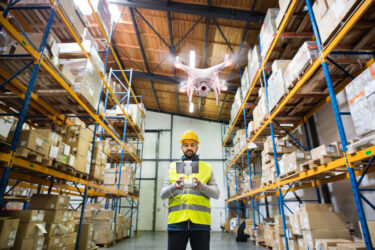
Transforming Operations with Drones: 10 Smart Drone Applications in Manufacturing

Speech Recognition and Beyond: 5 Practical Applications of NLP in Audio Processing
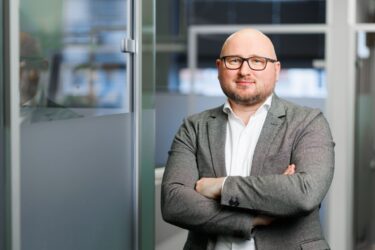
“Let’s Dive into Deep Tech” Newsletter by Przemysław Szleter
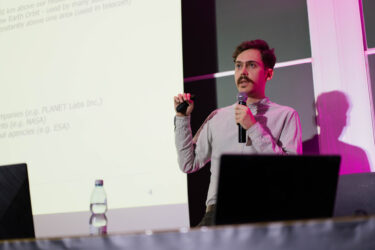
Satellite onboarding data processing – Michał Affek Warsaw IT Days
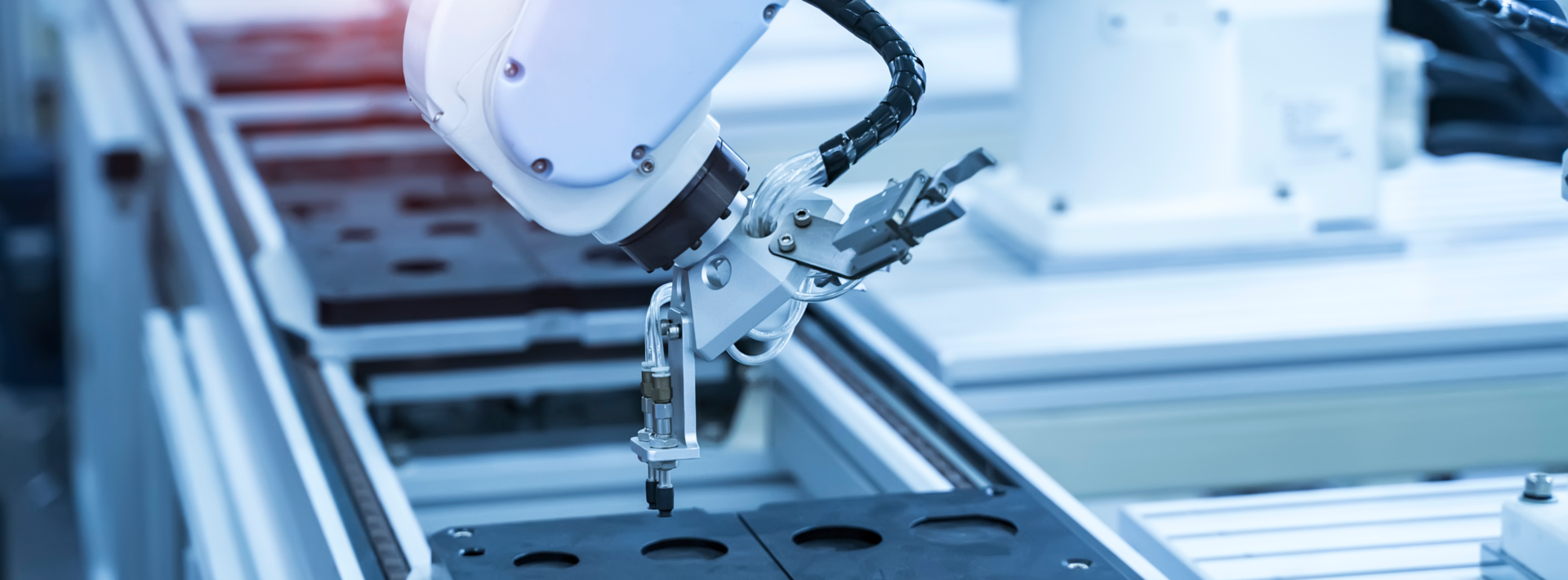
Read about our computer vision projects and achievements
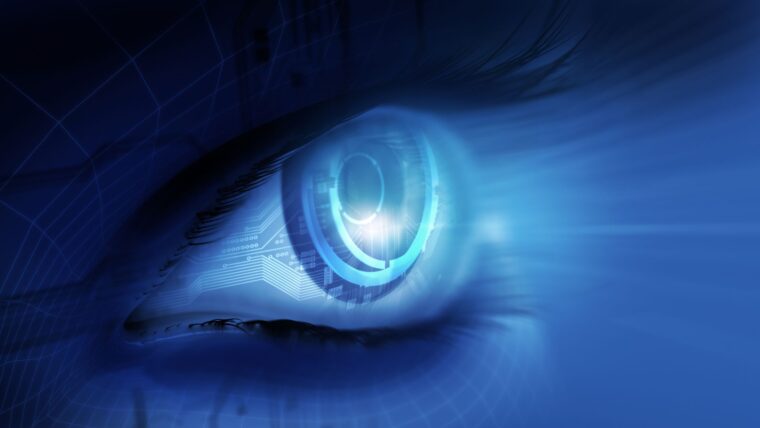
Gaze estimation in the wild. Creating a ground-breaking eye-tracking system for in-context market research

AI modeling and machine learning in detecting OA disease among dogs
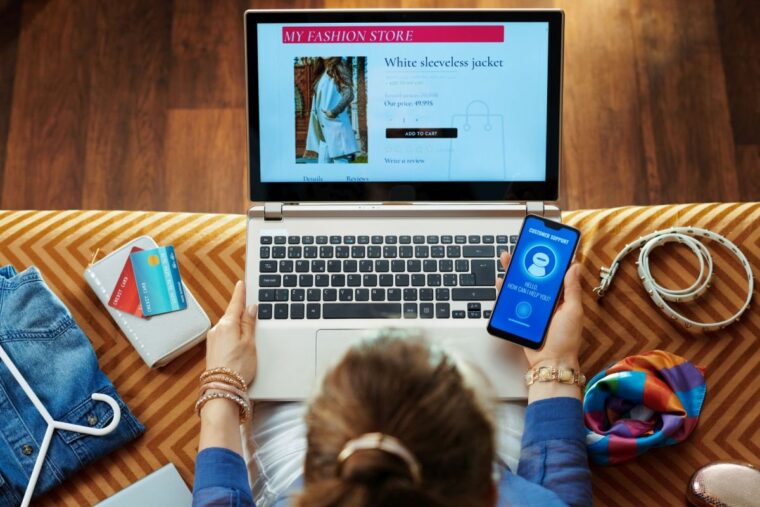
How an AI chatbot can transform e-commerce customer support
Our patents
One patent pending in Computer Vision field.
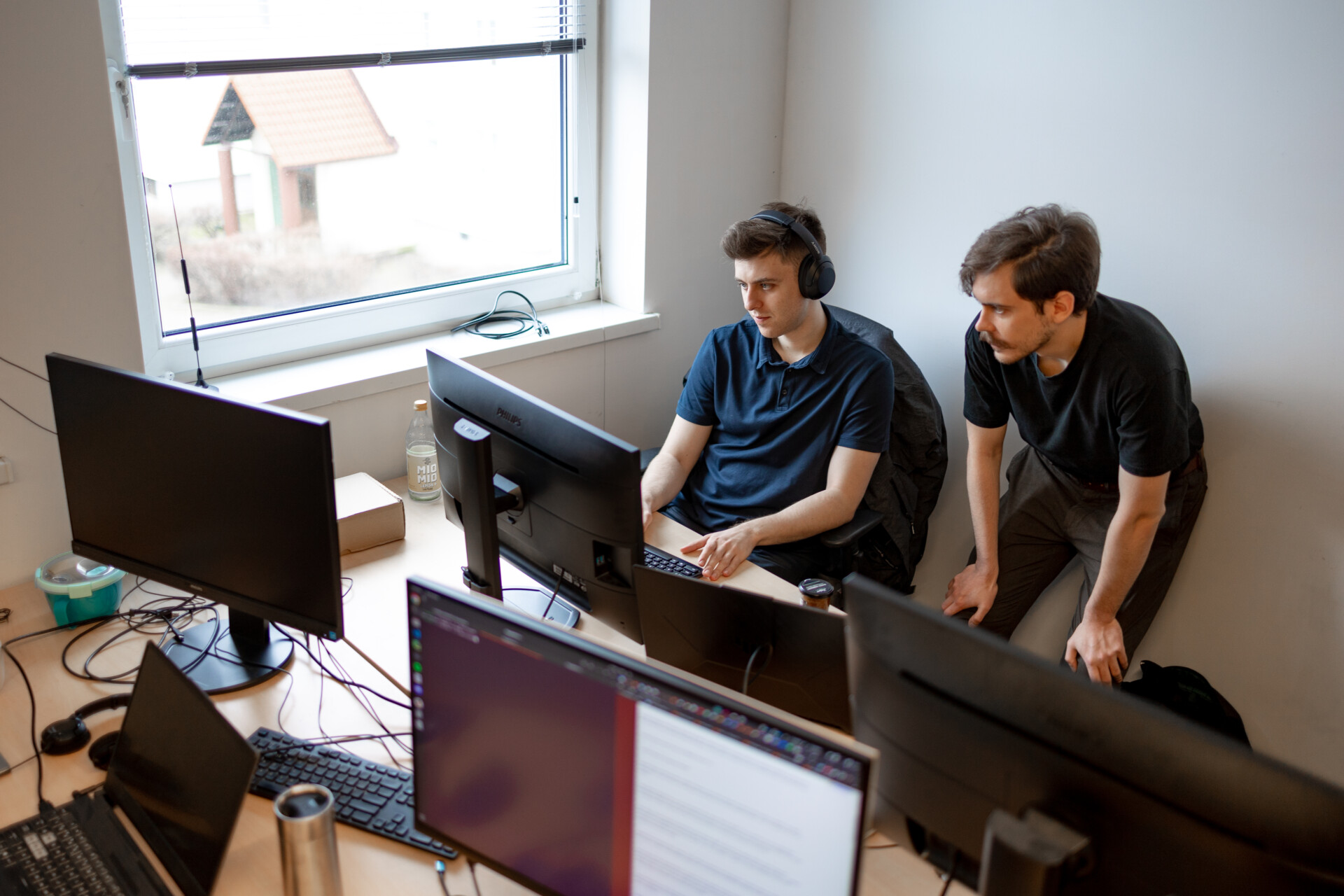
Build your next computer vision project with us.
Do you have an idea of how you could use computer vision for your next project? Our team of expert researchers and engineers is there for you to help you achieve your goals. Don’t be afraid of being bold. We can find a solution to different challenges. Let’s talk about it.